How AI Prevents Costly Solar Backup System Failures (Before They Happen)
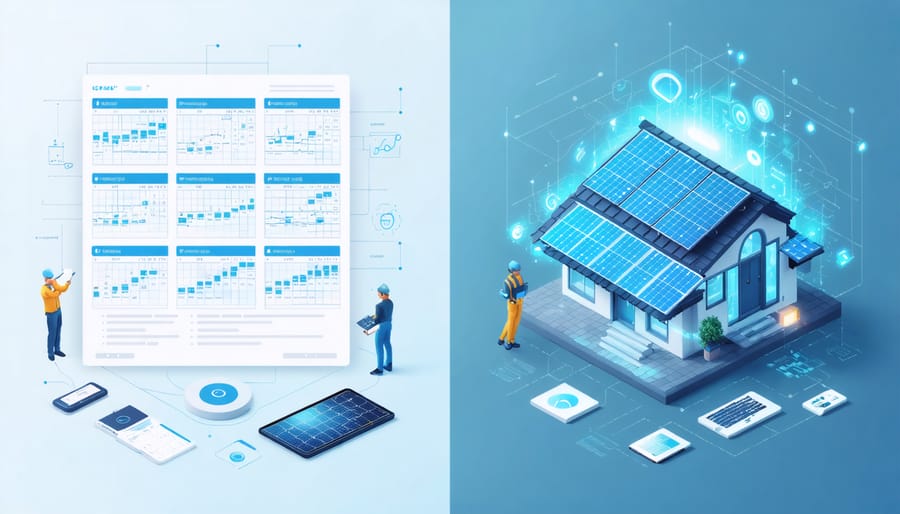
Transform your maintenance operations with AI-powered predictive systems that detect equipment failures before they occur, creating truly resilient energy systems for sustainable performance. By analyzing real-time sensor data from solar installations, these intelligent systems reduce downtime by up to 75% while cutting maintenance costs by 30%. European facilities implementing predictive maintenance have reported ROI within 12-18 months, primarily through prevented failures and optimized resource allocation.
Advanced machine learning algorithms now process thousands of data points per second, monitoring critical parameters like inverter efficiency, panel degradation, and weather impact patterns. This proactive approach enables maintenance teams to schedule interventions precisely when needed, eliminating both unnecessary inspections and unexpected breakdowns. For facility managers and technical directors, this represents a paradigm shift from reactive to predictive operations, ensuring maximum system uptime while optimizing maintenance budgets.
The integration of predictive maintenance into solar energy infrastructure has become a cornerstone of modern energy management, particularly in regions with ambitious renewable energy targets. This technology not only enhances system reliability but also extends equipment lifespan, making it an essential investment for forward-thinking organizations committed to sustainable energy solutions.
The Evolution of Solar Backup System Maintenance
From Regular Inspections to Smart Monitoring
Traditional maintenance approaches relied heavily on fixed schedules and reactive repairs, often leading to unnecessary downtime and missed early warning signs. Technicians would conduct routine inspections at predetermined intervals, regardless of the actual condition of solar equipment, resulting in both wasted resources and potential oversight of developing issues.
Modern predictive maintenance systems represent a significant leap forward, leveraging artificial intelligence and real-time sensor data to monitor system performance continuously. These smart solutions analyse multiple parameters simultaneously, from inverter efficiency to panel temperature variations, creating a comprehensive picture of system health. The AI algorithms learn from historical data patterns to identify potential failures before they occur, enabling precise intervention timing.
This evolution has transformed maintenance from a rigid, calendar-based activity into a dynamic, need-based process. European facilities implementing these systems report up to 30% reduction in maintenance costs and a 25% decrease in unexpected failures. The shift towards predictive maintenance aligns perfectly with the European Union’s Industry 4.0 initiatives, promoting increased automation and data exchange in manufacturing technologies while supporting sustainability goals through optimised resource utilisation.
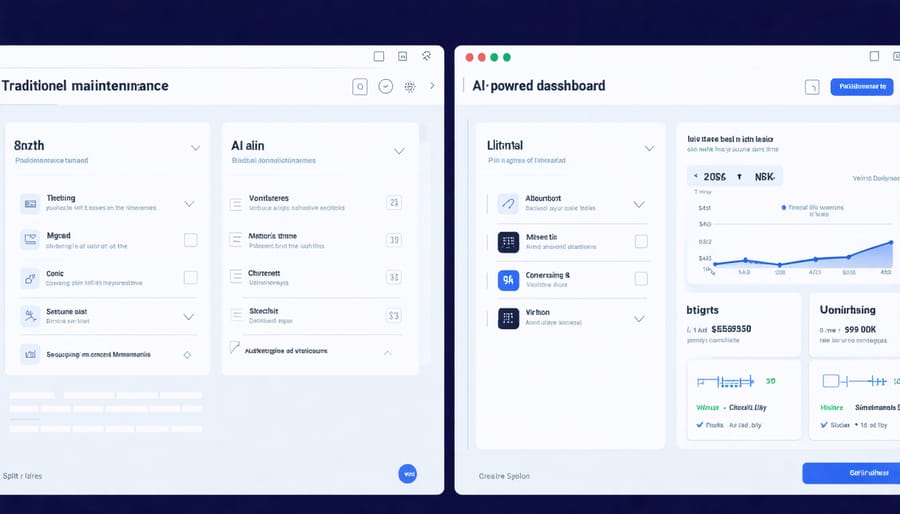
The Cost of Reactive Maintenance
Reactive maintenance can significantly impact your operational budget, with unexpected system failures leading to substantial financial losses. Studies show that European businesses lose an average of €50,000 per hour during critical system downtime, while unplanned maintenance typically costs 3-9 times more than scheduled interventions. These expenses extend beyond immediate repair costs, affecting your entire operation through reduced energy production, emergency labour charges, and potential damage to connected equipment.
Understanding your backup system costs becomes crucial when considering that reactive maintenance often requires premium-priced emergency parts and results in longer system downtimes. The ripple effect impacts productivity, customer satisfaction, and regulatory compliance, potentially leading to penalties in some European markets.
Moreover, the stress on system components during unexpected failures often shortens their overall lifespan, forcing earlier replacements and increasing your total cost of ownership. This reactive approach creates a cycle of emergency responses that strains both technical resources and maintenance budgets, making it an unsustainable long-term strategy for modern solar energy systems.
How AI Predictive Maintenance Works
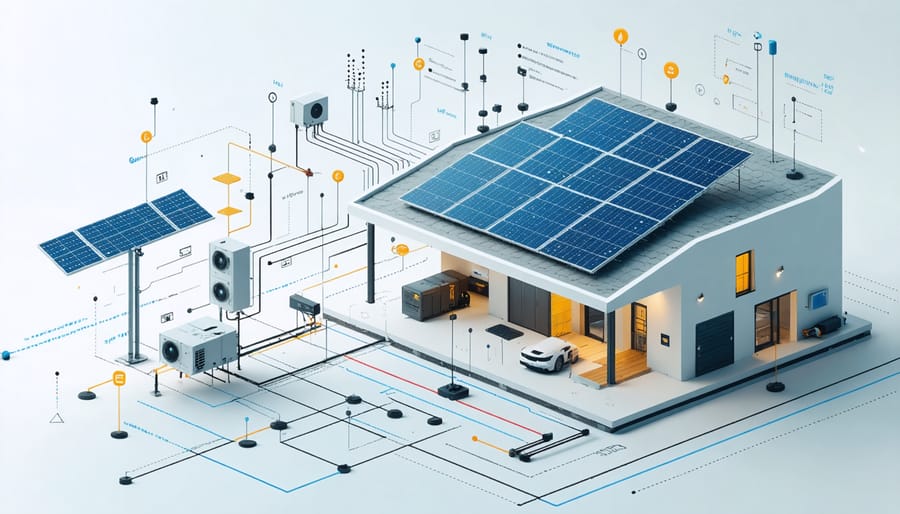
Real-Time Data Collection and Analysis
Modern predictive maintenance systems rely on sophisticated sensor networks that continuously gather vital operational data from solar power components. These smart monitoring systems collect multiple data points, including temperature variations, voltage fluctuations, current flows, and environmental conditions.
Advanced sensors equipped with Internet of Things (IoT) capabilities transmit real-time data to centralised processing units, where powerful algorithms analyse the information stream. This continuous monitoring enables the detection of subtle changes in performance patterns that might indicate potential issues before they become critical failures.
The data processing infrastructure typically employs edge computing architecture, allowing for immediate analysis at the source while sending filtered data to cloud servers for deeper analysis. This dual-layer approach ensures both rapid response to urgent issues and comprehensive long-term performance tracking.
Key parameters monitored include:
– Power output efficiency
– Inverter performance metrics
– Battery charge/discharge cycles
– Operating temperatures
– Weather conditions
– Grid interaction patterns
The system processes this information through multiple analytical layers, comparing current readings against historical benchmarks and manufacturer specifications. This creates a dynamic baseline for normal operation, making it possible to identify deviations that require attention while reducing false alarms through intelligent pattern recognition.
Pattern Recognition and Failure Prediction
Modern predictive maintenance systems employ sophisticated pattern recognition algorithms that continuously analyze data from multiple sensors across solar installations. These AI-driven systems learn to identify subtle variations in performance metrics, temperature patterns, and electrical signatures that often precede equipment failures.
By processing historical maintenance data alongside real-time measurements, the AI creates baseline performance models for each component. When current readings deviate from these established patterns, the system flags potential issues for investigation. For instance, the AI might detect slight changes in inverter efficiency or unusual temperature fluctuations in storage batteries weeks before they would become noticeable through conventional monitoring.
The system’s machine learning capabilities improve over time, becoming increasingly accurate at distinguishing between normal operational variations and genuine warning signs. This predictive approach enables maintenance teams to schedule interventions during planned downtime, reducing unexpected failures and maximizing system availability. For European solar installations, where weather patterns and seasonal variations can significantly impact performance, this intelligent pattern recognition has proven particularly valuable in maintaining optimal system efficiency year-round.
Automated Response Systems
Modern predictive maintenance systems incorporate sophisticated automated response protocols that activate when potential issues are detected. These responses occur in three distinct phases: alert generation, issue classification, and action implementation. When sensors detect anomalies in performance metrics or equipment behavior, the system immediately generates targeted alerts to relevant maintenance personnel.
The response mechanism utilises machine learning algorithms to classify issues based on severity and urgency. Critical problems trigger immediate system interventions, such as automatic shutdown procedures or load redistribution, while less urgent matters are scheduled for planned maintenance windows. This intelligent prioritisation ensures optimal resource allocation and minimises unnecessary downtime.
For solar energy installations, these automated systems can adjust panel positioning, modify power flow parameters, or initiate protective measures during adverse weather conditions. The response protocols are customisable to specific facility requirements and can be integrated with existing building management systems, creating a comprehensive maintenance ecosystem that operates with minimal human intervention while maintaining maximum system efficiency and reliability.
Through continuous learning and adaptation, these automated responses become increasingly refined, leading to more precise and effective maintenance interventions over time.
Implementation Benefits for European Solar Systems
Enhanced System Reliability
Predictive maintenance systems dramatically enhance the reliability of solar energy installations by identifying potential issues before they escalate into system failures. Through continuous monitoring and data analysis, these systems achieve remarkable improvements in uptime metrics, with many European facilities reporting availability rates exceeding 99%.
The impact on performance is equally significant. Advanced algorithms analyze operational patterns and environmental conditions to optimize maintenance schedules, resulting in a 15-30% reduction in unexpected downtime. This proactive approach ensures that solar installations operate at peak efficiency throughout their lifecycle.
Real-world implementations across Europe demonstrate compelling results. For instance, commercial solar installations using predictive maintenance systems report an average increase of 12% in system availability compared to traditional maintenance approaches. The systems also extend equipment lifespan by identifying stress factors and wear patterns early, allowing for timely interventions that prevent catastrophic failures.
Performance metrics show that predictive maintenance reduces mean time between failures (MTBF) by up to 45% while decreasing maintenance costs by 25-30%. This improvement in reliability directly translates to increased energy production and revenue generation. For residential installations, this means more consistent power supply and fewer disruptions to daily activities. Industrial users benefit from better planning capabilities and reduced operational risks, making solar energy an even more attractive investment for sustainable business operations.
Cost Optimization and ROI
The implementation of predictive maintenance systems presents a compelling financial case for businesses across Europe. Initial investment costs typically range from €50,000 to €200,000, depending on system scale and complexity. However, these systems consistently demonstrate strong return on investment through multiple cost-saving channels.
Studies show that predictive maintenance can reduce maintenance costs by 25-30% and eliminate up to 75% of breakdowns. For a medium-sized industrial facility, this translates to annual savings of €100,000-150,000 through decreased downtime, optimised spare parts inventory, and extended equipment lifespan.
The ROI calculation becomes particularly attractive when considering indirect benefits. Reduced emergency repairs, which often cost 3-5 times more than planned maintenance, contribute significantly to cost savings. Energy efficiency improvements of 5-15% are commonly reported, as equipment operates at optimal performance levels.
Most organisations achieve positive ROI within 12-18 months of implementation. A comprehensive cost-benefit analysis should consider:
– Reduced maintenance labour costs
– Decreased equipment replacement frequency
– Lower energy consumption
– Minimised production losses
– Improved workforce productivity
– Enhanced safety compliance
For maximum financial benefit, organisations should adopt a phased implementation approach, starting with critical assets and gradually expanding the system based on demonstrated success. This strategy allows for better resource allocation and helps build a strong business case for larger-scale deployment.
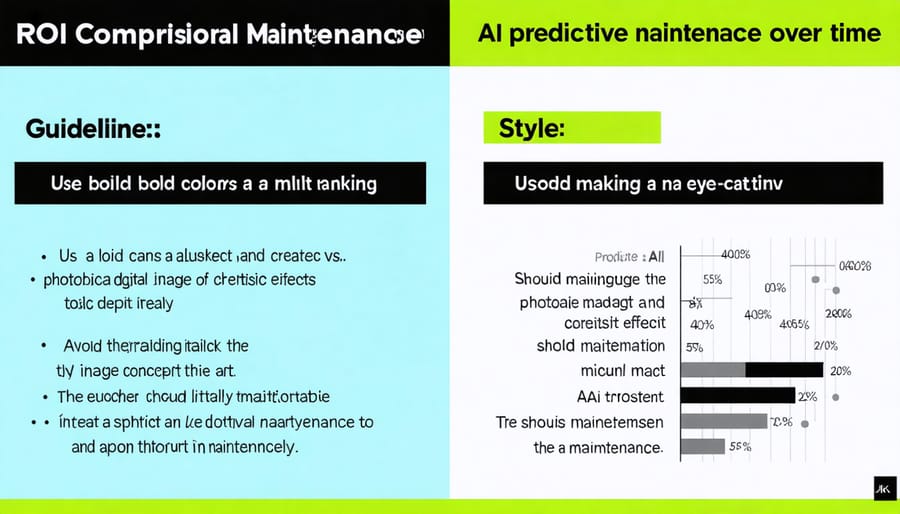
Future-Proofing Your Solar Investment
Integration with Smart Grid Systems
The integration of predictive maintenance systems with smart grid infrastructure represents a significant advancement in solar energy management. By connecting these systems to the broader energy network, operators can achieve unprecedented levels of operational efficiency and grid stability. This integration enables real-time data exchange between solar installations and grid operators, creating a more responsive and adaptive energy ecosystem.
Modern smart grid systems can leverage predictive maintenance data to optimize power distribution, balance loads, and anticipate potential system failures before they impact the grid. This capability is particularly valuable for future-proof solar installations that must maintain consistent performance despite varying weather conditions and demand patterns.
The integration process typically involves implementing standardized communication protocols, secure data transfer systems, and automated response mechanisms. These components work together to create a seamless flow of information between individual solar installations and central grid management systems. For European businesses and utilities, this integration offers substantial benefits, including reduced maintenance costs, improved grid stability, and enhanced energy trading capabilities.
Key advantages of this integration include:
– Automated performance optimization
– Enhanced grid stability through predictive load management
– Improved energy storage utilization
– Reduced maintenance-related downtime
– More efficient resource allocation for maintenance teams
As smart grid technology continues to evolve, these integrated systems will play an increasingly vital role in ensuring the reliability and efficiency of Europe’s renewable energy infrastructure.
Continuous Learning and Improvement
Predictive maintenance systems represent a dynamic technology that continuously evolves and improves through machine learning algorithms and data analysis. As these systems collect more operational data from solar installations, they become increasingly accurate in their predictions and maintenance recommendations.
The learning process occurs through multiple channels. First, the AI analyses patterns in equipment performance data, correlating various parameters with actual maintenance events and system failures. This creates an ever-expanding knowledge base that refines prediction accuracy over time. Second, feedback from maintenance technicians and system operators helps validate and adjust the AI’s recommendations, creating a virtuous cycle of improvement.
European solar facilities implementing these systems have reported significant improvements in prediction accuracy after just six months of operation. The systems typically start with 75-80% accuracy and can achieve over 95% accuracy within two years of deployment, depending on data quality and system complexity.
Modern predictive maintenance platforms also incorporate regular software updates that introduce new features and analytical capabilities. These updates often include improved algorithms, additional sensor integration options, and enhanced reporting capabilities, ensuring the system remains current with technological advancements.
For optimal results, organisations should establish clear processes for data quality management and regular system performance reviews. This approach ensures the AI continues to learn and adapt to changing operational conditions while maintaining high reliability in its predictions.
The adoption of AI-driven predictive maintenance systems represents a transformative step forward in solar energy system management. By implementing these innovative solutions, European businesses and homeowners can achieve substantial reductions in maintenance costs while significantly improving system reliability and performance. The data-driven insights provided by predictive maintenance not only prevent unexpected failures but also optimize maintenance schedules, ensuring maximum energy production efficiency throughout the year.
The benefits are clear and measurable: reduced downtime, extended equipment lifespan, and optimized maintenance resource allocation. Organizations implementing these systems typically report maintenance cost savings of 15-30% and increased system availability of up to 20%. For the European solar energy sector, this translates into enhanced competitiveness and improved sustainability metrics.
Looking ahead, the integration of predictive maintenance will become increasingly essential for maintaining competitive advantage in the renewable energy landscape. As AI technology continues to evolve, these systems will become more sophisticated, offering even greater precision in fault prediction and maintenance optimization. The investment in predictive maintenance today positions organizations at the forefront of solar energy innovation, ensuring long-term operational excellence and sustainable growth.
For businesses and homeowners considering solar energy solutions, embracing predictive maintenance is not just about staying current with technology – it’s about making a strategic investment in the future of their energy infrastructure. The time to adopt these solutions is now, as early implementers stand to gain the most significant competitive advantages in terms of operational efficiency and system reliability.
Leave a Reply