AI Energy Storage Management: The Hidden Power Costs You Need to Know
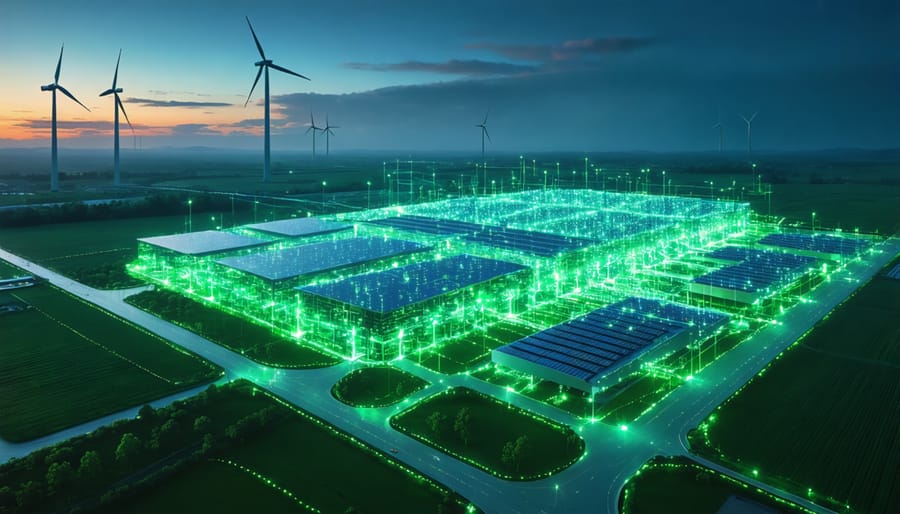
As artificial intelligence revolutionizes our energy landscape, its own power consumption presents a crucial sustainability challenge. Modern AI systems, particularly large language models and deep learning networks, consume substantial amounts of electricity – with some estimates suggesting that training a single advanced AI model can generate as much CO2 as five cars over their lifetimes. Yet paradoxically, AI also holds the key to optimizing energy storage management systems and reducing overall grid consumption.
European businesses implementing AI solutions must balance these competing realities: the technology’s energy footprint versus its potential to revolutionize energy efficiency. While data centers housing AI systems currently account for approximately 2% of global electricity usage, innovative cooling technologies and renewable energy integration are rapidly transforming how we power artificial intelligence. Understanding this dynamic is essential for organizations seeking to harness AI’s benefits while maintaining their commitment to sustainability.
The Real Energy Cost of AI in Storage Systems
Computing Power Requirements
The computational demands of artificial intelligence in energy storage systems are substantial and growing. Modern AI algorithms, particularly those managing complex storage networks, require significant processing power and data center capacity. A typical industrial-scale AI system for energy management processes millions of data points hourly, demanding anywhere from 100 to 1,000 kilowatt-hours per day of operational energy.
Storage requirements are equally demanding, with AI models needing extensive database capacity for historical performance data, weather patterns, and consumption trends. These systems typically require dedicated servers with high-performance computing capabilities, often consuming between 5-15 kW of continuous power.
The good news is that technological advancements are making AI systems more efficient. Edge computing solutions are reducing centralized processing needs, while optimised algorithms are delivering better performance with lower computational requirements. European data centers are increasingly adopting energy-efficient hardware and cooling systems, significantly reducing the overall power footprint of AI operations.
For businesses implementing AI-driven storage solutions, it’s crucial to factor in these computational requirements during the planning phase to ensure sustainable operation and optimal performance.
Data Center Impact
The rapid expansion of artificial intelligence applications has significantly impacted data center energy costs across Europe. Modern AI systems require substantial computational power for training and operation, leading to increased energy demands in data facilities. Recent studies indicate that a single large-scale AI model training session can consume as much electricity as 100 European households use in a year.
Data centers running AI storage management systems typically operate 24/7, requiring constant cooling and power supply. The energy intensity is particularly notable in deep learning applications, where complex algorithms process vast amounts of data continuously. European data centers managing AI operations now account for approximately 2.5% of the continent’s total electricity consumption, with this figure projected to rise significantly by 2025.
Innovation in energy-efficient computing and sustainable cooling systems offers promising solutions. Many facilities are implementing smart thermal management and adopting renewable energy sources to offset their environmental impact. This transformation towards sustainable AI infrastructure aligns with European Union’s green energy initiatives while maintaining operational efficiency.
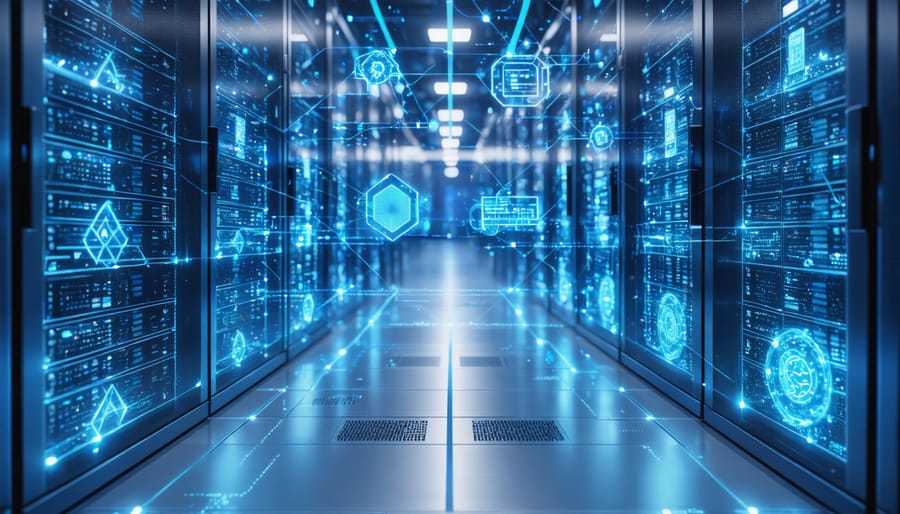
Balancing AI Benefits Against Energy Costs
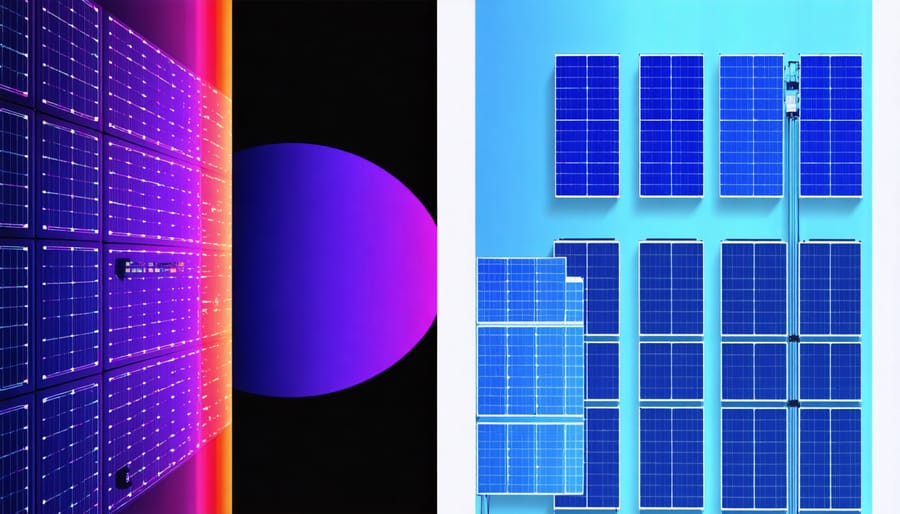
Optimization Advantages
While artificial intelligence systems require significant energy to operate, they simultaneously offer remarkable optimization advantages that can lead to net energy savings. AI algorithms excel at analyzing vast amounts of data to identify patterns and inefficiencies in energy storage systems, enabling smarter resource allocation and reduced waste.
In the European context, AI-powered systems have demonstrated up to 25% improvement in energy storage efficiency through predictive maintenance and intelligent load management. These systems can anticipate peak demand periods, optimize charging cycles, and reduce unnecessary energy transfers, resulting in substantial energy savings despite their operational requirements.
Machine learning algorithms continuously adapt and improve their performance, leading to increasingly efficient energy storage solutions over time. For instance, smart grid applications using AI have shown the ability to reduce storage losses by implementing advanced forecasting models that predict renewable energy generation and consumption patterns with remarkable accuracy.
The optimization extends beyond direct energy storage. AI systems can coordinate multiple storage units, balance grid loads, and integrate various renewable energy sources more effectively. This comprehensive approach to energy management helps offset the initial energy investment required to run AI systems, creating a positive energy balance in the long term.
For businesses and industries, these optimization capabilities translate into reduced operational costs and improved sustainability metrics, making AI-driven energy storage solutions an increasingly attractive investment despite their energy consumption requirements.
Net Energy Impact
When evaluating AI-managed energy storage systems, the net energy impact reveals a compelling efficiency story. While AI systems require significant computational power, their optimization capabilities typically generate energy savings that outweigh their consumption. Studies indicate that AI-managed storage systems can improve overall energy efficiency by 15-25% compared to traditional management methods.
The energy balance calculation considers three primary factors: direct AI computational energy usage, reduced storage losses through predictive management, and optimized energy distribution. Modern AI systems consume approximately 3-5 kWh per day to manage a medium-sized storage facility, but their smart algorithms can prevent up to 50 kWh of daily storage losses through precise charge-discharge cycles.
In practical terms, European facilities implementing AI-managed storage solutions report net positive energy impacts within the first three months of operation. For instance, a commercial storage facility in Germany documented a 20% reduction in total energy losses after implementing AI management, despite the additional computing requirements.
The key to achieving positive net energy impact lies in right-sizing the AI system to the storage capacity. Small-scale installations might benefit from edge computing solutions that consume minimal power, while larger facilities can justify more sophisticated AI implementations due to their proportionally higher optimization potential.
As AI technology continues to advance, these systems become increasingly efficient, further improving the energy balance in favor of implementation.
Sustainable Solutions for AI Energy Usage
Energy-Efficient Algorithms
Recent innovations in AI development have led to significant breakthroughs in energy-efficient algorithms, addressing the growing concern of AI’s environmental impact. These modern approaches focus on optimising computational processes while maintaining high performance levels, essential for sustainable AI implementation across European industries.
One notable advancement is the development of sparse neural networks, which reduce energy consumption by eliminating unnecessary connections within the network architecture. This approach can achieve up to 90% reduction in computational requirements while maintaining similar accuracy levels to traditional models.
Quantization techniques have emerged as another powerful tool for energy efficiency. By reducing the precision of numerical representations in AI models, organisations can significantly decrease memory usage and power consumption. This method has proven particularly effective in edge computing applications, where energy resources are often limited.
Knowledge distillation represents another innovative approach, where larger, energy-intensive models transfer their learned capabilities to smaller, more efficient networks. This “teacher-student” methodology enables the creation of lightweight models that maintain high performance while consuming substantially less energy.
Progressive learning systems are gaining traction, particularly in European industrial applications. These systems adapt their computational requirements based on task complexity, activating additional resources only when necessary. This dynamic approach ensures optimal energy usage while maintaining reliable performance levels.
The implementation of these energy-efficient algorithms has demonstrated that sustainable AI is not just an aspiration but a practical reality. European businesses adopting these optimised approaches can expect reduced operational costs while contributing to environmental sustainability goals, creating a win-win situation for both economic and ecological considerations.
Renewable Energy Integration
The integration of renewable energy sources with AI systems presents a compelling solution to address the growing energy demands of artificial intelligence infrastructure. By leveraging sustainable energy storage solutions and smart grid technologies, organizations can significantly reduce their AI operations’ carbon footprint while maintaining optimal performance.
Solar and wind energy systems, when coupled with advanced AI algorithms, create a symbiotic relationship where clean power generation meets intelligent consumption management. This integration enables dynamic load balancing, predictive maintenance, and efficient energy distribution across AI computing facilities. European data centers are increasingly adopting this approach, with many facilities now powered by a combination of solar arrays and wind farms.
The implementation of renewable energy for AI systems requires sophisticated energy management systems that can handle variable power generation and consumption patterns. Modern storage technologies, such as advanced battery systems and thermal storage, help bridge the gap between peak renewable energy generation and AI workload demands. This ensures consistent power supply while maximizing the use of clean energy sources.
Furthermore, AI itself plays a crucial role in optimizing renewable energy integration. Machine learning algorithms can predict weather patterns, adjust energy consumption based on availability, and manage storage systems for maximum efficiency. This creates a positive feedback loop where AI not only benefits from renewable energy but also enhances its effectiveness.
For European businesses implementing AI solutions, the combination of renewable energy sources with intelligent power management represents a sustainable path forward, aligning with both environmental goals and operational efficiency requirements.
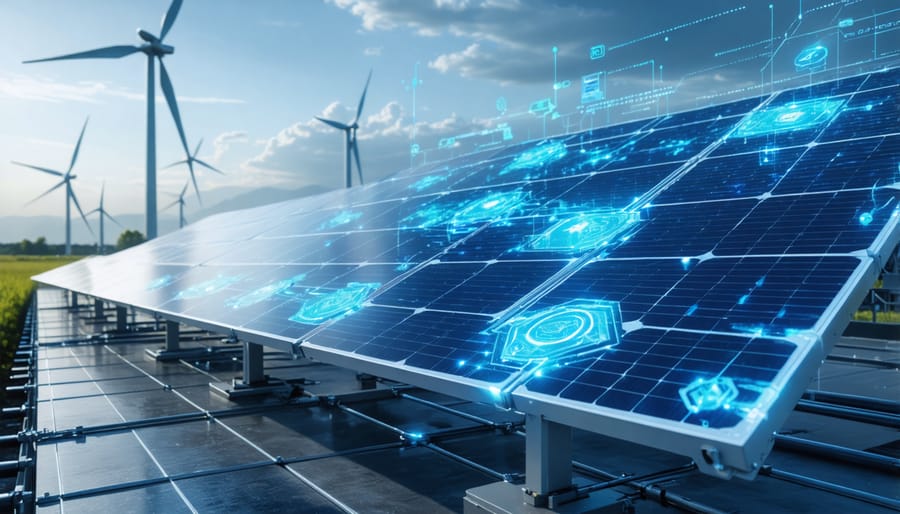
As we’ve explored throughout this article, the relationship between artificial intelligence and energy consumption presents both challenges and opportunities for European businesses and industries. While AI systems currently demand significant power resources, innovative solutions are emerging to address these concerns. The integration of renewable energy sources, particularly solar power, combined with smart grid technologies and efficient data center designs, offers promising pathways toward sustainable AI implementation.
Looking ahead, the future of AI energy consumption appears increasingly optimistic. Advancements in hardware efficiency, coupled with sophisticated energy management systems, are steadily reducing the environmental impact of AI operations. European initiatives leading the way in sustainable computing and green data centers demonstrate that responsible AI deployment is not only possible but economically viable.
For businesses considering AI implementation, the key lies in adopting a holistic approach to energy management. By combining AI capabilities with renewable energy solutions and smart storage systems, organizations can maximize efficiency while minimizing their environmental footprint. As technology continues to evolve, we can expect to see even more innovative solutions that further bridge the gap between artificial intelligence and sustainable energy consumption.
Leave a Reply